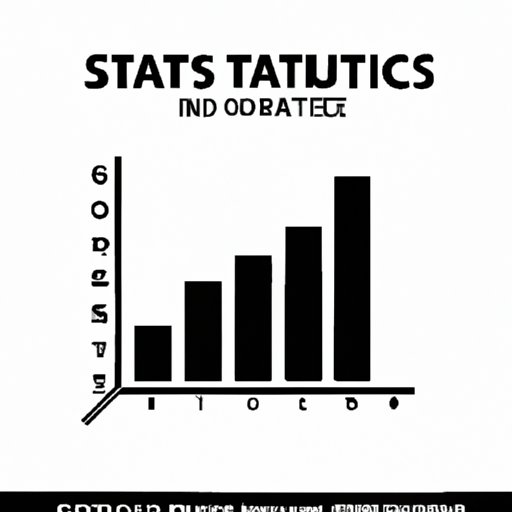
I. Introduction
Calculating test statistic is an essential part of statistical analysis that helps researchers to draw conclusions from their data accurately. Whether you’re a scientist or a student, understanding how to calculate test statistic is a skill that can have a big impact on your research. In this article, we offer a step-by-step guide to calculating test statistic for beginners and experts alike. We will simplify the process and provide techniques to maximize the statistical significance of the data insights gathered.
II. Mastering the Basics: A Step-by-Step Guide to Calculating Test Statistic
A test statistic is a numerical value that summarizes the data and allows us to determine if the data supports the hypothesis or not. It is calculated using the sample data and used to make inferences about the population.
To calculate a test statistic, the first step is to formulate the null hypothesis and the alternative hypothesis. The null hypothesis is usually that there is no difference in the population parameters. The alternative hypothesis is the opposite of the null hypothesis.
Next, we collect a sample of data from the population and calculate the necessary parameters such as mean, variance, and standard deviation. The test statistic is calculated using these parameters, and we compare it to the critical value to determine whether to reject or accept the null hypothesis.
For example, suppose we want to test whether the average height of a group of students in a college is different from the national average height. We first collect a sample of heights from the students. The null hypothesis is the average height of the students is the same as the national average and the alternative hypothesis is that the average height of the students is different from the national average. After calculating the sample mean, variance, and standard deviation, we can calculate the test statistic by subtracting the national average height from the sample mean, then dividing by the standard error.
The test statistic value is then compared to the critical value for the corresponding alpha level. If the test statistic is greater than the critical value, we reject the null hypothesis and conclude that the sample mean is different from the national average.
III. Understanding the Importance of Test Statistics in Statistical Analysis
Test statistics play a critical role in statistical analysis, particularly in hypothesis testing. They provide a way to compare our sample data to the population parameters and determine if our findings are statistically significant.
By using test statistics, we can estimate the population parameters from the sample data and determine if our sample is representative or an outlier. Furthermore, test statistics help us to identify factors that affect our data’s variability, which enables us to make better predictions about the population.
For example, suppose we want to determine if a new drug is effective in reducing cholesterol levels. We would collect data from a group of participants, including their cholesterol level before and after taking the drug. By calculating the test statistic, we can determine if the drug significantly reduced the cholesterol levels. This information can be used to recommend the drug to others with high cholesterol.
IV. Simplifying the Process: How to Calculate Test Statistic Without Any Complex Formulas
Calculating test statistic can be complex and time-consuming, especially when dealing with a large dataset. However, there are several quick and easy methods to calculate a test statistic without relying on complex formulas.
One simplified approach is to use software packages such as Microsoft Excel to perform the calculations automatically. Excel has built-in formulas that calculate the test statistic, critical values, and p-values in a fraction of the time compared to the manual calculations.
Another approach is to use statistical tables, such as z-table and t-table, to determine the critical value for the corresponding alpha level. These tables provide an easy reference to find the critical value and eliminate the need for any complex calculations.
By using these simplified methods, we can save time while still providing reliable and accurate results.
V. Maximizing Your Data Insights: A Primer on Calculating Test Statistics for Beginners
For beginners, calculating test statistics may be intimidating. However, with a solid foundation of statistical concepts, anyone can master this skill easily.
The first step is to understand the different types of test statistics available, such as the t-test and z-test. The t-test is used when the sample size is small, while the z-test is used when the sample size is large.
Next, it’s essential to understand how to interpret the results of a test statistic. The test statistic is compared to the critical value from the distribution. If the test statistic is larger than the critical value, we can conclude that the sample data is statistically significant and supports the alternative hypothesis.
For example, suppose we want to test whether a new product increases sales. The null hypothesis is that the new product does not increase sales and the alternative hypothesis is that the new product increases sales.
After collecting the sample data, the researcher calculates the test statistic and compares it to the critical value for the corresponding alpha level. If the test statistic is larger than the critical value, we can conclude that the new product statistically increases sales.
VI. Unlocking the Power of Statistical Significance: Techniques for Calculating Test Statistic
Statistical significance is an important factor when analyzing data. It indicates how confident we can be in the results we have obtained and conclusions we have drawn. To maximize statistical significance, we can use several techniques when calculating test statistics.
One technique is to increase the sample size. A larger sample size can reduce variability and increase the power of the statistical test. Another technique is to control the experimental conditions to ensure that the data is uniform. This can reduce the possibility of confounding factors that could skew the results.
Lastly, we can use more advanced statistical tests, such as the ANOVA test or chi-square test, which provides more robust results by comparing multiple groups simultaneously.
By using these techniques, we can unlock the power of statistical significance and obtain more meaningful results from our data analysis.
VII. A Comprehensive Approach: From Theory to Practice in Calculating Test Statistic for Any Data Set
Calculating test statistic for complex data sets can be challenging. However, by following a comprehensive approach, we can simplify the process and obtain reliable results.
The first step is to identify the type of data set we are analyzing, such as continuous or categorical data. The next step is to choose the appropriate statistical test, such as the t-test or ANOVA test, based on the data type and research question.
We can then collect the sample data and calculate the necessary parameters, such as mean, variance, and standard deviation, for the corresponding statistical test. The test statistic is calculated using these parameters and compared to the critical value to determine the statistical significance of the data.
For example, suppose we want to test whether there’s a significant difference in the mean score between two groups of students who participated in different teaching methods. We would first identify the data as continuous and choose the t-test as the appropriate statistical test.
The next step is to collect the sample data, calculate the mean, variance, and standard deviation for each group, and then calculate the test statistic by subtracting the mean of one group from the other and dividing by the standard error of the difference. The test statistic is then compared to the critical value for the corresponding alpha level to determine statistical significance.
VIII. Conclusion
Calculating test statistics is a crucial part of statistical analysis that helps us to draw accurate conclusions from our data. By mastering the basics, we can gain valuable insights into our data and make data-driven decisions.
When calculating test statistics, it’s essential to choose the appropriate statistical test, simplify the process, and understand the results we obtain fully. By doing so, we can unlock the power of statistical significance and obtain reliable results.
For those looking to improve their test statistic skills, we recommend practicing with real-life examples and seeking the assistance of statistical experts if necessary.