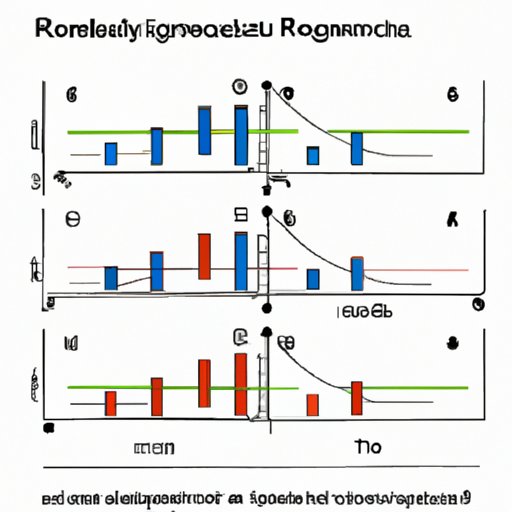
Introduction
If you’re a student, researcher, or professional working with data, you’ve probably heard of statistical measures like standard deviation, range, and mean. Another important statistical measure that is often used to understand the spread of data is the interquartile range. In this article, we will provide a step-by-step guide on how to find the interquartile range, its practical applications, visual interpretations, real-life cases, and a comparative analysis with other statistical measures.
Step-by-Step Guide
The interquartile range is the range of values between the first and third quartiles of the data set. The first quartile is the value that splits the bottom 25% of data from the top 75%, while the third quartile splits the top 25% from the bottom 75%.
For example, let’s say we have the following data set:
12, 18, 20, 22, 27, 30, 33, 36, 40, 44, 50, 55
To find the first and third quartiles, we need to first order the data set from smallest to largest:
12, 18, 20, 22, 27, 30, 33, 36, 40, 44, 50, 55
The median of the entire data set is between the 6th and 7th values, which are 30 and 33. Therefore, the median is (30 + 33) / 2 = 31.5. This value splits the data set into two halves.
Next, we find the median of the lower half of the data set (12, 18, 20, 22, 27, 30). The median of the lower half is between the 2nd and 3rd values, which are 18 and 20. Therefore, the median is (18 + 20) / 2 = 19.
Finally, we find the median of the upper half of the data set (33, 36, 40, 44, 50, 55). The median is between the 3rd and 4th values, which are 40 and 44. Therefore, the median is (40 + 44) / 2 = 42.
The first and third quartiles are 19 and 42, respectively. To calculate the interquartile range, we simply subtract the first quartile from the third quartile:
Interquartile range = third quartile – first quartile
Interquartile range = 42 – 19 = 23
The interquartile range in this example is 23. This value tells us that 50% of the data lies within the range of 19 and 42.
It’s important to note that the interquartile range is not affected by outliers, which makes it a useful tool for understanding the spread of data in skewed distributions.
Practical Application
The interquartile range is used in various fields such as finance, healthcare, and quality control. Understanding the interquartile range in these fields is essential as it provides insight into the spread of data and helps inform decisions.
In finance, the interquartile range is used to calculate risk and returns of investment portfolios. Investors may use the interquartile range to determine the level of risk associated with different investments. Higher interquartile ranges imply higher levels of risk, while lower interquartile ranges indicate lower levels of risk.
In healthcare, the interquartile range is used to understand the spread of different kinds of data such as patients’ weight, age, and income. Understanding the interquartile range can help hospital administrators understand the spread of health data and adapt their services to meet patients’ needs.
In quality control, the interquartile range is used to detect outliers and measure the variability of data. Quality control managers may use the interquartile range to determine if an organization’s production processes are functioning correctly and to identify opportunities for improvement.
Visual Interpretation
Boxplots, also known as box-and-whisker plots, provide a visual representation of the interquartile range. In a boxplot, the box itself represents the interquartile range, with the line in the middle of the box representing the median. The whiskers represent the range of data outside the upper and lower quartiles, while outliers are represented as individual points.
Here’s an example of a boxplot:
As you can see, the box itself represents the interquartile range. This box spans from the first quartile to the third quartile, with the line in the middle representing the median. The whiskers show the range of data outside the interquartile range, while outliers are shown as individual points.
It’s important to note that boxplots can be misleading if the data set is too small or too skewed. In some cases, histograms or other visual representations may be a better option to represent the data set.
Real-Life Cases
The interquartile range has been used in many real-life situations to understand the spread of data and solve specific problems. Here are some examples:
Real-Life Case #1: Studying the Wealth Gap in Mexico
Researchers studying the wealth gap in Mexico used the interquartile range to divide the population into four groups based on wealth levels. The researchers found that the median household income in the top group was 33 times higher than in the bottom group, indicating a large wealth gap in the country. By understanding the interquartile range, the researchers were able to provide empirical evidence on the magnitude of the wealth gap in Mexico.
Real-Life Case #2: Identifying Air Pollution in Beijing
Researchers studying air pollution in Beijing used the interquartile range to understand the concentration of particulate matter in the city. By calculating the interquartile range, the researchers were able to identify areas where the air pollution concentrations were highest, and recommended public health interventions in those areas.
Real-Life Case #3: Reducing Infant Mortality Rates in India
Researchers studying infant mortality rates in India used the interquartile range to understand the spread of factors that contribute to infant mortality, such as maternal age, education, and weight. By calculating the interquartile range, the researchers identified a significant correlation between maternal education and infant mortality rates. This finding has led to policy changes to improve maternal education levels in India.
Comparative Analysis
While the interquartile range is an important statistical measure, it’s not always the best measure to use in all situations. Other measures of statistical dispersion, like the standard deviation and range, are also commonly used.
The standard deviation, for example, measures how spread out a data set is from the mean. The range measures the difference between the highest and lowest values of a data set. While these measures are useful, they both have limitations. The standard deviation is heavily influenced by outliers, while the range doesn’t provide any information about the middle 50% of the data.
The interquartile range is preferred in situations where the data set is skewed or contains outliers. It provides a measure of the spread of the middle 50% of the data, making it a useful tool for understanding the spread of data in a variety of situations.
Conclusion
The interquartile range is an important statistical measure that provides insights into the spread of data in a variety of fields, including finance, healthcare, and quality control. By understanding how to calculate the interquartile range, its practical applications, visual interpretations, real-life cases, and comparative analysis with other statistical measures, you’ll have a powerful tool to make informed decisions based on data.